Continuation of metallic solutions using preblur
The MaxEnt formalism has the tendency of producing spurious noise around the \(\omega=0\) point. This is usually not problematic for insulating solutions, but not desirable for metallic spectral functions.
One strategy to tackle this is the so-called preblur technique [1]. There, a so-called hidden image \(H\) is introduced, with the spectral function being \(A = B H\) [2]. The matrix \(B\) is the blur matrix; multiplying by \(B\) is equal to a convolution with a Gaussian (its width \(b\) is an external parameter). Then, in the cost function, both \(H\) and \(A\) are used,
The parametrization \(H(v)\) is, e.g., Bryan’s parametrization, \(H(v) = D e^{Vv}\). The parametrization \(A(H)\) is, of course, \(A = B H\).
The class MaxEntCostFunction
accepts a parameter A_of_H
.
Only two different parametrizations for \(A(H)\) are implemented: the IdentityA_of_H
and PreblurA_of_H
variants.
The former just chooses the usual \(A(H) = H\), the latter uses \(A(H) = BH\) as discussed here.
Note that, for performance reasons, the kernel has to be redefined to include the blur matrix. This is done by choosing the PreblurKernel
.
The calculation should be performed using different values for \(b\). The best value of \(b\) can be determined similarly to determining \(\alpha\); e.g., by calculating the probability of every \(b\) or by looking at \(\log(\chi^2)\) of \(\log b\).
Example
A full example:
1
2from triqs_maxent.analyzers.linefit_analyzer import fit_piecewise
3
4try:
5 # TRIQS 2.1
6 from triqs.gf import *
7 GfImFreq
8except NameError:
9 # TRIQS 1.4
10 from triqs.gf.local import *
11from triqs_maxent import *
12from triqs_maxent.analyzers.linefit_analyzer import fit_piecewise
13
14# whether we want to save and plot the results
15save = False
16plot = True
17
18# construct a test Green function
19# for reference, G(w)
20G_w = GfReFreq(window=(-3, 3), indices=[0])
21G_w << SemiCircular(1)
22
23# the G(iw)
24G_iw = GfImFreq(beta=40, indices=[0], n_points=50)
25G_iw << SemiCircular(1)
26
27# we inverse Fourier-transform G(iw) to G(tau)
28G_tau = GfImTime(beta=G_iw.beta, indices=[0], n_points=102)
29G_tau.set_from_fourier(G_iw)
30# and add some noise (MaxEnt does not work without noise)
31G_tau.data[:, 0, 0] = G_tau.data[:, 0, 0] + \
32 1.e-4 * np.random.randn(len(G_tau.data))
33
34tm = TauMaxEnt()
35# the following allows to Ctrl-C the calculation and choose what to do
36tm.interactive = True
37tm.set_G_tau(G_tau)
38# set an appropriate alpha mesh
39tm.alpha_mesh = LogAlphaMesh(alpha_min=0.08, alpha_max=1000, n_points=30)
40tm.set_error(1.e-4)
41
42# run without preblur
43result_normal = tm.run()
44last_result = result_normal
45
46K_tau = tm.K
47
48# loop over different values of the preblur parameter b
49results_preblur = {}
50for b in [0.05, 0.1, 0.15, 0.2, 0.25, 0.3, 0.4]:
51 print('Running for b = {}'.format(b))
52 tm.A_of_H = PreblurA_of_H(b=b, omega=tm.omega)
53 tm.K = PreblurKernel(K=K_tau, b=b)
54 # initialize A(w) with the last result, this leads to faster convergence
55 tm.A_init = last_result.analyzer_results['LineFitAnalyzer']['A_out']
56 results_preblur[b] = tm.run()
57 last_result = results_preblur[b]
58
59# save the results
60if save:
61 from h5 import HDFArchive
62 with HDFArchive('preblur.h5', 'w') as ar:
63 ar['result_normal'] = result_normal.data
64 ar['results_preblur'] = [r.data for r in results_preblur]
65
66# extract the chi2 value from the optimal alpha for each blur parameter
67chi2s = []
68# we have to reverse-sort it because fit_piecewise expects it in that order
69b_vals = sorted(list(results_preblur.keys()), reverse=True)
70for b in b_vals:
71 r = results_preblur[b]
72 alpha_index = r.analyzer_results['LineFitAnalyzer']['alpha_index']
73 chi2s.append(r.chi2[alpha_index])
74
75# perform a linefit to get the optimal b value
76b_index, _ = fit_piecewise(np.log10(b_vals), np.log10(chi2s))
77b_ideal = b_vals[b_index]
78print('Ideal b value = ', b_ideal)
79
80if plot:
81 import matplotlib.pyplot as plt
82 from triqs.plot.mpl_interface import oplot
83 oplot(G_w, mode='S')
84 result_normal.analyzer_results['LineFitAnalyzer'].plot_A_out()
85 results_preblur[b_ideal].analyzer_results['LineFitAnalyzer'].plot_A_out()
86 plt.xlabel(r'$\omega$')
87 plt.ylabel(r'$A(\omega)$')
88 plt.legend(['original', 'normal', 'preblur'])
89 plt.xlim(-2.5, 2.5)
90 plt.savefig('preblur_A.png')
91 plt.show()
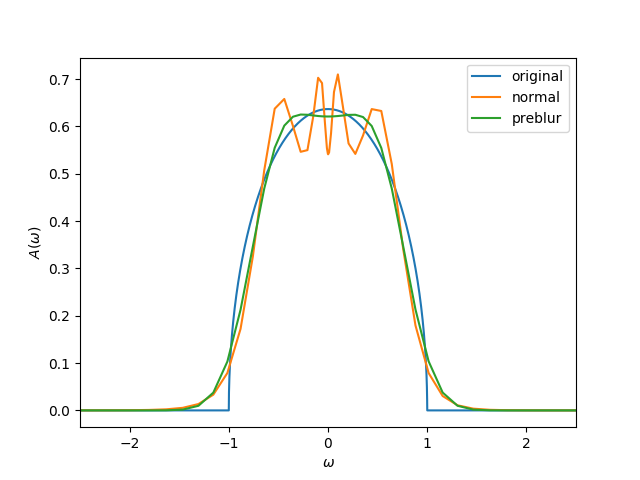
Footnotes